Making Learning Part of Life
Beyond the “Gift Wrapping” Approach to Technology
Gerhard Fischer
Center for LifeLong Learning & Design (L3D)
Department of Computer Science and
Institute of Cognitive Science
University of Colorado, Boulder
Note: These notes are based on a presentation at the NSF Symposium “Learning & Intelligent Systems,” June 26th, 1996. They are structured in accordance with the major questions and issues discussed at the symposium. The author would like to thank
- the organizers of this symposium for the opportunity to present the ideas and the research efforts behind them documented in these notes, and
- his collaborators at CU Boulder, specifically the members of the L3D Center who contributed in the development of the ideas behind these notes.
Feedback to these notes would be greatly appreciated: ().
More information about the L3D Center can be found at: http://l3d.cs.colorado.edu/
Table of Contents
- The State of Relevant Knowledge
- Important Frontiers for Fundamental Interdisciplinary Research
- Building a Successful Interdisciplinary Investigation
- Responses of the Relevant Science, Engineering Research, and Education Communities
- Long-Term Societal Impacts of This Research
Introduction. There is general agreement as we approach the next century and next millennium that our society is changing into a knowledge and information society. We will face new opportunities and new challenges in all dimensions of our lives. But the future is not out there to be “discovered”: It has to be invented and designed. A research agenda on “Learning and Intelligent Systems” must focus on “making learning a part of life,” and the implications this has on how‹under the influence of new media, new social structures, and new objectives for a quality of life‹human beings will think, create, work, learn, and collaborate in the future.
1. The State of Relevant Knowledge
1.1 Learning: Current Theories
Current trends in educational theory make the following fundamental assumptions about learning (arguments supporting this view can be found in the books by L. Resnick “Knowing, Learning and Instruction”, D. Norman “Things That Make Us Smart”, and M. Csikszentmihalyi “Flow”):
- Learning is a process of knowledge construction, not of knowledge recording or absorption.
- Learning is knowledge-dependent; people use their existing knowledge to construct new knowledge.
- Learning is highly tuned to the situation in which it takes place.
- Learning needs to account for distributed cognition requiring knowledge in the head to combined with knowledge in the world.
- Learning is affected as much by motivational issues as by cognitive issues.
1.2 Lifelong Learning
Lifelong Learning: A Ubiquitous Goal. Lifelong learning has emerged as one of the major challenges for the worldwide knowledge society of the future. A variety of recent events support this claim: (1) 1996 is the “European Year of Lifelong Learning,” (2) UNESCO has included “Lifetime Education” as one of the key issues in its planning, and (3) the G7 group of countries has named “Lifelong Learning” as a main strategy in the fight against unemployment. Despite this great interest, there are few encompassing efforts to tackle the problem in a coherent way. Lifelong learning cannot be investigated in isolation by looking just at one small part of it, such as K-12 education, university education or worker re-education.
Learning as a New Form of Labor. The previous notions of a divided lifetime-education followed by work-are no longer tenable. Learning can no longer be dichotomized, spatially and temporally, into a place and time to acquire knowledge (school) and a place and time to apply knowledge (the workplace). Professional activity has become so knowledge-intensive and fluid in content that learning has become an integral and inseparable part of “adult” work activities. Professional work can no longer simply proceed from a fixed educational background; rather, education must be smoothly incorporated as part of work activities fostering growth and exploration. Similarly, children require educational tools and environments whose primary aim is to help cultivate the desire to learn and create, and not to simply communicate subject matter divorced from meaningful and personalized activity. Lifelong learning is a continuous engagement in acquiring and applying knowledge and skills in the context of authentic, self-directed problems. L3D’s theoretical framework for lifelong learning is grounded in descriptive and prescriptive goals such as: (1) learning should take place in the context of authentic, complex problems (because learners will refuse to quietly listen to someone else’s answers to someone else’s questions); (2) learning should be embedded in the pursuit of intrinsically rewarding activities; (3) learning-on-demand needs to be supported because change is inevitable, complete coverage is impossible, and obsolescence is unavoidable; (4) organizational and collaborative learning must be supported because the individual human mind is limited; and (5) skills and processes that support learning as a lifetime habit must be developed.
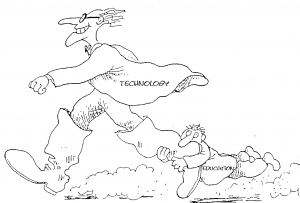
Figure 1: Education & Technology
1.3 Lifelong Learning and Design
Lifelong learning integrates and mutually enriches the cultures of work and education. Central to this vision in our own research is the notion of design activity, a model of work that is open-ended and long-term in nature, incorporates personalized and collaborative aspects, and combines technical and aesthetic elements. Design is an argumentative process, involving ongoing negotiations and trade-offs; it is also a collaborative process making increasing use of new social structures brought about by the advent of computer networks and “virtual communities.” The communality that crucially binds these and other design activities together is that they are centered around the production of a new, publicly accessible artifact. Engineers and architects design infrastructure and buildings, lawyers design briefs and cases, politicians design policies and programs, educators design curricula and courses, and software engineers design computer programs. It is impossible for design processes to account for every aspect that might affect the designed artifact. Therefore design must be treated as an evolutionary process in which designers continue to learn new things as the process unfolds. The relationship between learning and design provides the impetus for the work done at the L3D Center. Because design is an essential aspect of all problem-solving activity, and since designers are constantly learning and communicating with each other, the research done at the L3D Center seeks to ground educational theory within the domain of technology that supports design and communication.
1.4 Beyond the “Gift Wrapping” Approach of Educational Reform-Rethinking, Reinventing, and Reengineering Education
A deeper understanding and more effective support for lifelong learning will contribute to the transformation that must occur in the way our society works and learns. A major finding in current business reengineering efforts is that the use of information technology had disappointing results compared to the investments made in it (see for example the book by Tom Landauer “The Trouble with Computers”). While a detailed causal analysis for this shortcoming is difficult to obtain, it is generally agreed that a major reason is that information technologies have been used to mechanize old ways of doing business—rather than fundamentally rethinking the underlying work processes and promoting new ways to create artifacts and knowledge.
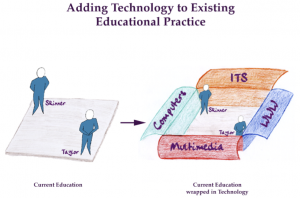
Figure 2: The “Gift Wrapping” Approach
We claim that a similar argument can be made for current uses of technology in education: it is used as an add-on to existing practices rather than a catalyst for fundamentally rethinking what education should be about in the next century. For example, the “innovation” of making transparencies available on the World-Wide Web (WWW) rather than distributing copies of them in a class takes advantage of the WWW as an electronic information medium. This may change the economics of teaching and learning, but it contributes little to introducing new epistemologies. “Old” frameworks, such as instructionism, fixed curriculum, memorization, decontextualized learning, etc., are not changed by technology itself. This is true whether we use computer-based training, intelligent tutoring systems, multimedia presentations, or the WWW. As Figure 1 shows, education often follows on the boot heels of technology rather than guiding the appropriate development and use of technology.
We need computational environments to support “new” frameworks for education such as lifelong learning, integration of working and learning, learning on demand, authentic problems, self-directed learning, information contextualized to the task at hand, (intrinsic) motivation, collaborative learning, and organizational learning. Figure 2 illustrates the “gift-wrapping “approach in which technology is merely wrapped around old frameworks for education. Figure 3 indicates what is needed instead: a richer conceptual framework, leading not just to the addition of technology but to the weaving of technology into learning and working.
Figure 3: Rethinking & Reinventing Education
Figure 4 tabulates the major changes required. It shows strong similarities between the behaviorist learning theory of B.F. Skinner and the models of industrial work of F.W. Taylor, and contrasts these with the lifelong approach to learning.
Skinner/Taylor | ……. | L3D |
---|---|---|
there is a “scientific”, best way to learn and to work (programmed instruction, computer-assisted instruction, production lines, waterfall models) |
→ | real problems are ill-defined and wicked; design is argumentative, characterized by a symmetry of ignorance among stakeholders |
separation of thinking, doing, and learning | → | integration of thinking, doing, and learning |
task domains can be completely understood | → | understanding is partial; coverage is impossible |
objective ways to decompose problems into standardizable actions | → | subjective, situated personal interests; need for iterative explorations |
all relevant knowledge can be explicitly articulated | → | much knowledge is tacit and relies on tacit skills |
teacher/manager as oracle | → | teacher/manager as facilitator or coach |
operational environment: mass markets, simple products and processes, slow change, certainty | → | customer orientation, complex products and processes, rapid and substantial change, uncertainty and conflicts |
2. Important Frontiers for Fundamental Interdisciplinary Research
2.1 Myths and Misconceptions
The current debate about the ability of computation and communication to fundamentally change education are (in our opinion) based on a number of fundamental myths and misconceptions. The most prevalent ones are:
- Computers by themselves will change education—There is no empirical evidence for this assumption based on the last 30 years of using computers to change education (such as computer-assisted instruction, computer-based training, or intelligent tutoring systems). Technology is no “Deus ex machina” taking care of education. As mentioned before, making slides available over the World-Wide Web rather than giving paper copies to students can be valuable, but will not change education. Instructionist approaches are not changed by the fact that information is disseminated by an intelligent tutoring system.
- Information is a scarce resource —“Dumping” even more decontextualized information on people is not a step forward in a world where most of us already suffer from too much information. Instead, technology should provide ways to “say the ‘right’ thing at the ‘right’ time in the ‘right’ way.” In our research, we have explored problems associated with high-functionality applications (such as operating systems, word processors, spreadsheets, etc.). Our empirical findings (which are universally true for all systems) are illustrated in Figure 5. These systems provide challenging problems for a research agenda for “Learning and Intelligent Systems,” because if future “progress” is achieved only by extending D4 to D4’, there will be no benefits for users. Instead of increasing the tool mastery burden of users even more, we need new concepts such as learning-on-demand, information delivery, and task-based unfolding, so users can incrementally explore and master such systems according to their needs.
The rectangle (D4) represents the actual information space of a system and the ovals represent users’ knowledge about the system’s information space. D1 represents concepts well known and easily employed by the users. D2 contains concepts known vaguely and used only occasionally often requiring passive help systems. D3 represents concepts users believe to exist in the system some of which lie outside the actual information space. In the case of increased functionality (as illustrated by D4′) the area D4-D3 (representing the functionality users are not even aware of) increases to D4’-D3 not that of the ovals. |
Figure 5: Levels of Users’ Knowledge About a System’s Information Spaces
- The content, value, and quality of information and knowledge is improved just because it is offered in multi-media or over the WWW Media itself does not turn irrelevant or erroneous information into more relevant information (as indicated by Figure 6). We must create innovative technologies (such as simulations, visualizations, critiquing, etc.) to let people “experience” knowledge in new ways.
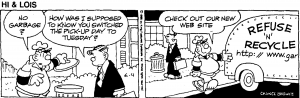
Figure 6: The Existence of Information Alone is Not Good Enough
- “Ease of use” is the greatest challenge or the most desirable goal for new technologies—Usable technologies that are not useful for the needs and concerns of people are of no value. Rather than assuming people should and will be able to do everything without a substantial learning effort, we should design computational environments that provide a low threshold for getting started and a high ceiling to allow skilled users to do the things they want to do.
- The “Nobel Prize winner” myth: Every school child will have access to a Nobel Prize winner—This was one of the selling points for the information superhighway. While this argument is true (or will be true soon) at the level of technical connectivity, it is doubtful that Nobel Prize winners will look forward to getting a few thousand e-mail messages a day.
- The single or most important objective of computational media is reducing the cost of education—Although we should not ignore any opportunity to use technology to lessen the cost of education, we should not lose sight of an objective that is of equal if not greater importance: increasing the quality of education.
- Human learning is equal to machine learning—Although we have deepened our understanding of human learning through progress in machine learning, there are fundamental dimensions, such as motivation and competing requirements for a human’s time, that make human learning a much more complex and interwoven activity than machine learning. There is substantial empirical evidence that the chief impediments to learning are not cognitive. It is not that students cannot learn; it is that they are not well motivated to learn.
2.2 Hypotheses and Requirements for Computational Environments Supporting Lifelong Learning
H1: User-directed and supportive. In any computational system supporting lifelong learning, the choice of tasks and goals (including the learning opportunities offered) must be under the control of the user/learner, and support must be contextualized to the user’s task.
H2: Contextualized presentation. A system supporting lifelong learning must present information to the user in a way that is maximally relevant to the user’s chosen project or task.
H3: Breakdowns as opportunities for learning. A system supporting lifelong learning will be sufficiently open-ended and complex that users will encounter breakdowns. The system must provide means for allowing users to understand, extricate themselves, and learn from breakdowns. Rather than attempting to eliminate trouble, the system should help users manage troubles and exploit breakdowns as opportunities rather than failures.
H4: End-user modification and programmability. A system supporting lifelong learning must provide means for significant modification, extension, and evolution by users.
H5: Supporting a range of expertise. Systems supporting lifelong learning will be employed over long periods of time by their users; hence, these systems must be able to accommodate users at progressively different levels of expertise.
H6: Useful and usable. Many existing research efforts and computational environments reflect an implicit belief and are grounded in a design philosophy that there is an inevitable design tradeoff between the notions of usefulness and usability. Systems supporting lifelong learning must be useful and usable.
H7: Promoting collaboration. Systems supporting lifelong learning must include means for collaboration among users.
2.3 The Grounding of Learning and Intelligent Systems in Theories of Learning
Technologies Transcending the “Gift-Wrapping” Approach. The grounding of technologies in new theories of learning (as briefly summarized in section 1.1) will contribute to transcend the “gift-wrapping” approach as shown by the following attributes of learning environments:
- Learning is a process of knowledge construction —requiring environments in which learners can be active designers and contributors rather than passive consumers. Research in end-user programming and end-user modifiability contributes toward this goal.
- Learning is knowledge-dependent —requiring environments supporting user-tailored information presentations such as differential descriptions of new information (for example: if someone wants to learn HTML and knows MS-WORD, the explanations and examples provided should be different than those given to a learner who knows Framemaker).
- Learning is highly tuned to the situation in which it takes place —requiring environments which are domain-oriented and which support human problem-domain interaction and not just human computer interaction. The information spaces presented and the information provided should be made relevant to the task at hand, something which computational media can achieve, but which is impossible for paper and pencil technologies.
- Learning needs to account for distributed cognition —requiring environments which create and define new role distributions between humans and computers. Most of what any individual “knows” today is not in her or his head, but is out in the world (e.g., in other human heads or embedded in media).
- Learning is affected as much by motivational issues as by cognitive issues —requiring environments which let people experience and understand why they should learn and contribute something. For example, learning-on-demand lets users access new knowledge in the context of actual problem situations and delivers information about which they are unaware in the context of their problem situations. Environments must allow users to take pride in their contributions and be awarded for them.
From Knowledge Aquisition to Knowledge Construction and Evolution: The Seeding, Evolutionary Growth and Reseeding (SER) Model. Most intelligent systems (including systems in support of learning such as Intelligent Tutoring Systems and Expert Systems) of the past have been developed as “closed” systems. The basic assumption was that during design time, a domain could be modeled completely by bringing domain experts (designers) and environment developers (knowledge engineers) together and the knowledge engineers would acquire the relevant knowledge from the domain experts and encode it into the system. This approach fails for the following reasons: (1) as argued before, much knowledge is tacit and only surfaces in specific problem situations; and (2) the world changes, and intelligent systems modeling this world must change accordingly. In our research, we have developed a process model to address these problems (see Figure 7). It postulates three major phases:
A seed will be created through a participatory design process between environment developers and domain designers. It will evolve in response to its use in new design projects because requirements fluctuate, change is ubiquitous, and design knowledge is tacit. Postulating the objective of a seed (rather then a complete domain model or a complete knowledge base) sets this approach apart from other approaches in intelligent systems development and emphasizes evolution as the central design concept.
Evolutionary growth takes place as learners use the seeded environment to undertake specific projects. During these design efforts, new requirements may surface, new components may come into existence, and additional design knowledge not contained in the seed may be articulated. During the evolutionary growth phase, the environment developers are not present, making end-user modification a necessity rather than a luxury. World-wide communities of practice can participate in this process if the WWW becomes an information environment for collaboration and sharing rather than one for information dissemination.
Reseeding, a deliberate effort of revision and coordination of information and functionality, brings the environment developers back to collaborate with domain designers to organize, formalize, and generalize knowledge added during the evolutionary growth phases. Organizational concerns play a crucial role in this phase. For example, decisions have to be made as to which of the extensions created in the context of specific design projects should be incorporated in future versions of the generic design environment. Drastic and large-scale evolutionary changes occur during the reseeding phase.
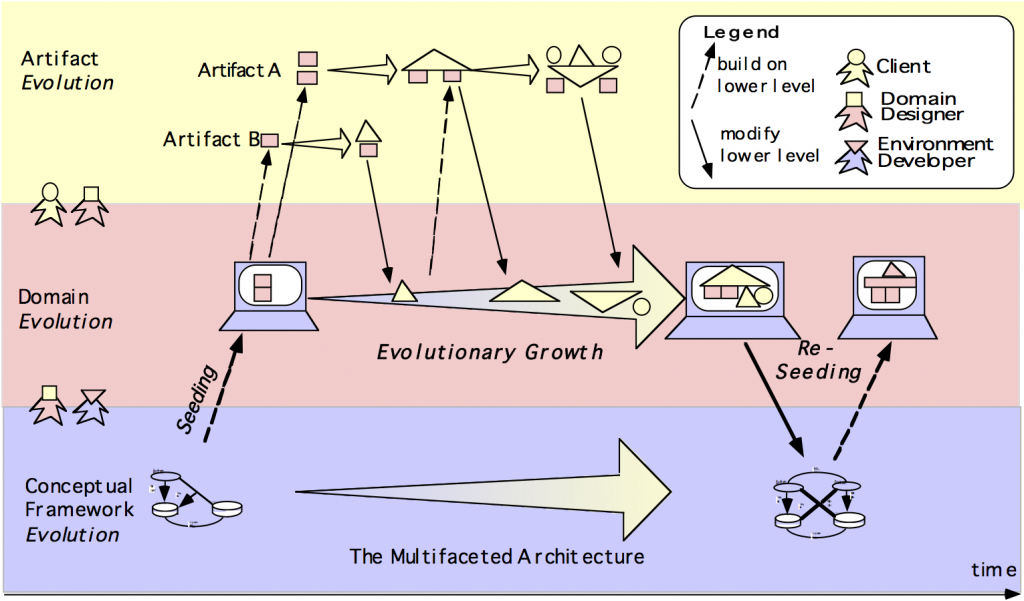
Figure 7: The SER Model: A process model for the development and
evolution of domain-oriented intelligent system
3. Building a Successful Interdisciplinary Investigation
Building successful interdisciplinary investigations is not a small task in a world in which specialization necessarily increases and the days of the universally educated “Renaissance Scholars” belong to the past. C.P. Snow, in his famous book “The Two Cultures,” identified the difficulty of “literary intellectuals” and “natural scientists” communicating successfully with each other. He claimed to have found a profound mutual suspicion and incomprehension, which had damaging consequences for the prospects of applying technology to the alleviation of the world’s problems. Many more different cultures exist today, e.g., novices versus skilled workers, software developers versus software users, industry people versus academics, and committed technophiles versus determined technophobes.
Experiences. At CU Boulder we have tried for the last ten years to build bridges among different cultures (the most relevant ones will be briefly mentioned):
- The Institute of Cognitive Science at CU Boulder brings together researchers from the humanities, the social sciences, the natural sciences, and engineering, acknowledging that problems the scientific community needs to address do not always fall neatly into the structures of established departments.
- In the context of university/industry relationships, we have tried to reinvent the purposes of such collaborations (and have explored research issues in detail in our close collaboration with NYNEX University and NYNEX Science and Technology).
- By working with the Boulder Valley School District and with several specific schools, we have tried to understand the problems of empowering teachers to become lifelong learners and of introducing and sustaining technology in school settings.
- Acknowledging that learning is desired and takes place outside formal institutions, we have recently started a collaboration with the Boulder County Healthy Community Initiative, a group of several hundred concerned citizens, which reflects on the future of our county.
- In our L3D Center we have brought together researchers and students from various parts of the world to understand different perspectives how people think about our world.
Our focus on lifelong learning and design has served as a forcing function to create these interdisciplinary investigations and they in return have been of critical importance to our understanding of the challenges of learning and intelligent systems.
Challenges. The building of successful interdisciplinary investigations faces the following challenges:
- To regard the existing “symmetry of ignorance” (a concept articulated by Rittel, who argues that among all the carriers of knowledge for any real problem there is nobody who has a guarantee that her or his knowledge is superior to any other person’s knowledge) as an opportunity rather than as a limitation or an undesired obstacle.
- To overcome the boundaries of creating divisions between basic and applied research by doing basic research on real problems.
- To find ways and to develop means to allow different cultures to talk to each other and to engage them as active participants in inventing the future (e.g., to liberate social scientists from their passive consumer and Cassandra role, and to make technologists aware that technological changes and innovations do not happen in isolation but in existing social networks involving people).
- To make sure that in multidisciplinary approaches to learning and intelligent systems all relevant disciplines are contributing (e.g., one might argue that at this time the design of learning and intelligent systems can profit more from research of social psychologists on matters of motivation, creativity, role models, etc. than from neuropsychology).
4. Responses of the Relevant Science, Engineering Research, and Education Communities
4.1 Challenges
“Making Learning a Part of Life” creates many challenges, requiring creative new approaches and collaboration among many different stakeholders. For illustration, just a few of them will be mentioned here.
- The educated and informed citizen of the future: ‘super-couch potato’ consumers or enlightened designers—The major innovation that many powerful interest groups push for with the information superhighway is to have a future where everyone shows her or his creativity and engagement by selecting one of at least 500 TV channels with a remote control. The major technical challenge derived from this perspective becomes the design of a “user-friendly” remote control. Rather than serving as the “reproductive organ of a consumer society” (Illich), educational institutions must fight this trend by cultivating “designers,” i.e., by creating mindsets and habits that help people become empowered and willing to actively contribute to the design of their lives and communities. This goal creates specific challenges for computational artifacts, such as the support of end-user programming and authoring.
- The “basic skills” debate—If the hypothesis that most job-relevant knowledge must be learned on demand is true, we have to ask ourselves: What is the role of “basic skills”? If, for example, the use of software packages dominates the use of mathematics in the workplace, shouldn’t a new function of mathematics education be teaching students to use these mathematical artifacts intelligently? Another important challenge is that the “old basic skills” such as reading, writing, and arithmetic, once acquired, were relevant for the duration of a human life; modern “basic skills” (tied to rapidly changing technologies) will change over time.
- Can we change motivation?—As mentioned, there is substantial empirical evidence that the chief impediments to learning are not cognitive but motivational. This raises the challenge of whether we can create learning environments in which learners work hard, not because they have to, but because they want to. We need to alter the perception that serious learning has to be unpleasant rather than personally meaningful, empowering, engaging, and even fun. In our research efforts we have developed computational environments to address these motivational issues; for example, our systems have explored making information relevant to the task at hand, providing challenges matched to current skills, creating communities (among peers, over the net), and providing access to real practitioners and experts.
- School-to-work transition—If the world of working and living (a) relies on collaboration, creativity, definition, and framing of problems; (b) deals with uncertainty, change, and distributed cognition; (c) copes with symmetry of ignorance; and (d) augments and empowers humans with powerful technological tools, then the world of schools and universities needs to prepare students to function in this world. Industrial-age models of education and work (based on Skinner and Taylor, as illustrated above) are inadequate to prepare students to compete in the knowledge-based workplace. A major objective of our lifelong learning approach is to reduce the gap between school and workplace learning. Our research addresses some of the major “school-to-work” transition problems and develops answers to the following questions:
-
- How can schools prepare learners and workers for a world that relies on interdependent, distributed, non-hierarchical information flow and rapidly shifting authority based on complementary knowledge?
- What “basic skills” are required in a world in which occupational knowledge and skills become obsolete in years rather than decades?
- How can schools (which currently rely on closed-book exams, the solving of given problems, and so forth) be changed so that learners are prepared to function in environments requiring collaboration, creativity, problem framing, and distributed cognition?
- To what extent will lifelong learning and new approaches to learning and teaching—such as learning on demand, learning while working, relations, and the involvement of professionals in schools—prepare learners for work?
4.2 What’s Wrong With Current Universities
We consider the self-application of our theories a critical element (and a unique opportunity) in the assessment of our research efforts. Universities as institutions need to be in the middle of rethinking the future of working and learning—applying their findings not only to other institutions, but to themselves. Using the previously developed framework causes us to critically examine our own work as university faculty members in the following ways:
- Understanding learning as active knowledge construction rather than passive knowledge absorption questions the dominance of lectures.
- Allowing learners to engage in authentic, self-directed learning activities is at odds with micro-managed curricula.
- Acknowledging that problem solving in the real world includes problem framing calls into question the practice of asking students to solve mostly given problems.
- Recognizing that most interesting problems in the real world do not have right or wrong answers, but instead must be solved by satisfying objectives that are most important for that situation.
- Acknowledging that the individual human mind is limited and that outside of schools people rely heavily on information and knowledge distributed among groups of people and various artifacts (distributed cognition) questions the value of closed-book exams, and requires a much greater emphasis on collaborative learning and communication skills.
5. Long-Term Societal Impacts of This Research
Research in learning and intelligent systems, especially if we want to move beyond the “gift wrapping” approach of technology, will have fundamental long-term societal impacts. It will force us to reinvent how we think, work, learn, create, and collaborate. It will change
- Institutions, e.g.,
- universities (as argued above)
- companies will have to become learning organizations
- Individuals, e.g.,
- who will have a desire to become independent of high-tech scribes in personally meaningful and important activities
- who would like to contribute to their (computer-enriched) reality rather than merely interacting with it
- Mindsets, e.g.
- teachers should see themselves not as truth-tellers and oracles, but as coaches, facilitators, learners, and mentors engaging with learners
- breakdowns and symmetry of ignorance need to be understood as opportunities
- Connections and collaborations, e.g.,
- connecting in new ways (e.g., distributed communities of practice and interest) will go along with disconnecting in old ways (being physically together, increased specialization)
- organizational learning supported by organizational memories will complement individual learning.
This research will provide us with opportunities to explore fundamentally new possibilities and limitations of computational media as they complement existing media. It will force us to think about new concepts such as sustainable communities of practice. It will pose the question of how large complex information spaces can be evolved over long periods of time, not by their professional designers but by their affected users. It will enrich the notion of distributed cognition, allowing us to draw different lines between what humans should do and what machines should do.
One may argue that our current thinking does not address the potential magnitude of the change. Have we arrived at a point where the change is of a similar magnitude to the time when our society moved from an oral to a literary society (and Socrates and Plato were arguing about the trade-offs associated with this change) or when Gutenberg’s printing press eliminated the scribes and gave everyone the opportunity to become literate? The fact that societies have often overestimated change in the short run and underestimated it in the long run suggests that we should make every effort to understand the long-term societal impacts of learning and intelligent systems.
Conclusion. As argued at the beginning, the future of how we live, think, create, work, learn, and collaborate is not out there to be “discovered”— it has to be invented and designed. Computational and communication media (firmly grounded in a deep understanding of theories and prescriptive goals) will be a critical force in shaping this future. Learning and intelligent systems research will play an important role in creating this future.